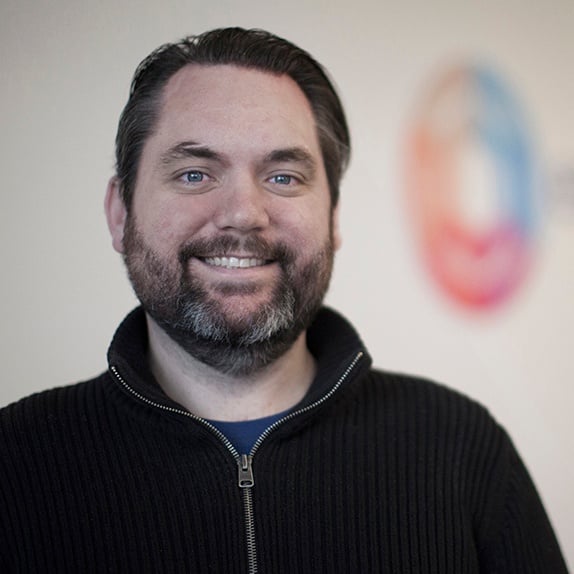
Everything is derivative. Take advantage of that. “New” ideas are the next step in an extensive network of existing people and ideas. If we can get the data and reconstruct the network, we can analyze it and understand where branches of a network have the potential for innovation.
Great ideas do not need to be created. They can be discovered.
Ideas are Networks
An astronomer starts chatting with a neuroscientist about the sheer amount of radio telescope data that neither he nor any of his colleagues know what do with. Through their conversation they discover that million-mile “slices” of space across hundreds of light-years is surprisingly similar to millimeter slice data of the neurons of the human brain. So why not use an existing MRI visualization to look at telescope data?
Though imperfect, it gives the astronomer the ability to “fly around” the supernova event the same way a surgeon can “walk around” the patient’s brain scans before operating.
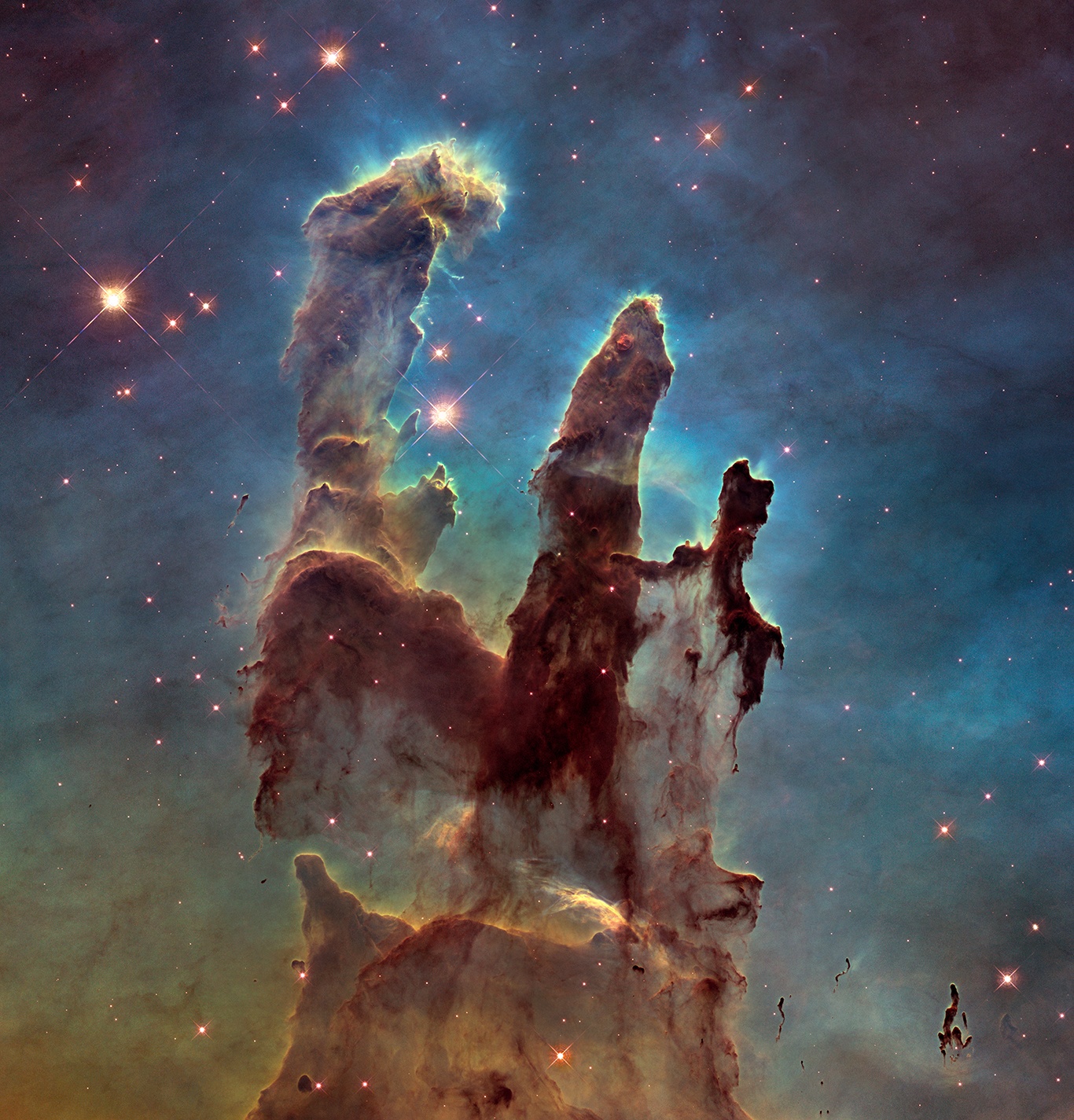
This is the famous Pillars of Creation nebula. Through MRI, astronomers learned that they were not pillars at all and acquired a better understanding of the velocity of galactic movement.
The outcome of this encounter was to launch a greater endeavor within the university toward inter-disciplinary study between astronomy and medicine, and in short order a familiar thing happened.
This is a cardiology map of arteries surrounding the heart showing thickness and pliability by color.
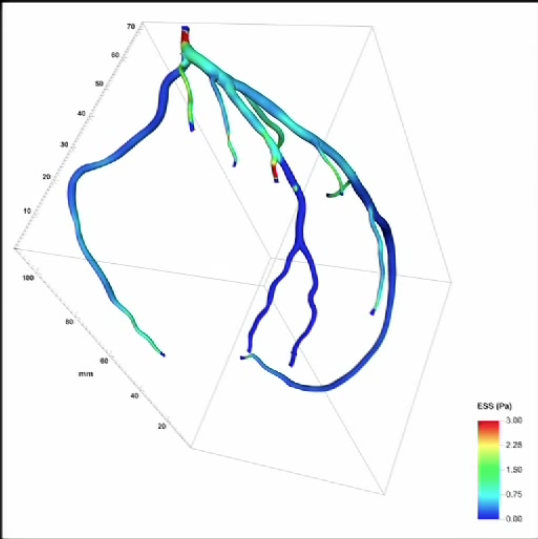
Medical researchers wanted to understand relationships between the branches of the arteries. They used ideas that came from multi-galactic astronomy meant to differentiate boundaries between multiple merging galaxies to create a new visualization. This new approach increased diagnosis rates of a particular disease from 39% to 62%.
But that idea came from geneticists, who used the technique to understand how different gene expression regions interrelate. And that idea came from evolutionary biology -- Darwin’s thinking about the interrelationships of speciation. From cardiology connecting back to astronomy, genetics, and evolutionary biology, they were solving different problems but not entirely, and they needed an understanding of where their problems overlapped.
This is not an isolated incident. The world is taking notice that ideas are networks, and that innovations often involve adapting ideas from adjacent domains.
We Need Not Rely on Serendipity
This chain of insight I just described was fortuitous. The people involved stumbled over some impactful aha-moments by literally stumbling into each other coincidentally. This phenomenon, however, can be orchestrated.
What’s required is to disassemble and rebuild networks of people, their interests, and their innovations into a visible network. Then we can analyze that network and mine it for the fundamental elements of those innovations. We can do this ahead of time, instead of just enjoying serendipity as an anecdote.
Unpacking and Visualizing the Parts of the Network
There are three basic elements that we’ve found we must unpack to capture the innovation potential of a network: the people, their attributes and the artifacts of their work. Attributes are what we call a person’s expertise, experience, affiliations, and other characteristics. It’s important to differentiate people and their attributes from artifacts of actual work, like a data set, an algorithm, or a particular analytical approach, for example. And you need to understand all three to really take advantage of a network.
You won’t get far just building a network of artifacts. New ideas don’t exist without the human minds that interpret and apply them. We’ve yet to find that humans can be eliminated from the recipe for innovation.
You’ve also got to know who those people are -- their attributes. Otherwise, you’re assembling people at random, without any hints at how they might relate to each other.
Without the artifacts, though, you’ve just got a group of people networking. They’re meandering through conversation hoping for an exciting realization. Artifacts are the basis for spotting an actual breakthrough and pursuing it.
Here’s one visualization of such a network. There are people with areas of expertise, and there are posters, representing the actual work they are doing - the techniques they are using and the data involved.
Building an analyzable structure of people, their interests, and their work product, all as first-class citizens, transcends a mere social network and becomes what we refer to as a cognitive network. In a cognitive network, researchers and technologists find untapped ways to collaborate around related work.
Analyzing the Overlap in the Network
Too much overlap isn’t disruptive enough. Two astronomers could never have innovated the way the neuroscientist and astronomer did. They’d more likely just continue having the same problem, never questioning its seeming inevitability.
Too little overlap is chaotic. Put a data scientist like me in conversation with a lawyer, with no context as to what we might have in common, and I’ll just end up telling lawyer jokes. The likelihood of insight is too low without some critical mass of overlap.
We conducted this kind of project with the Gates Foundation's Next Generation Scientist program. They bring together researchers from all over the world to work on a number of medical issues in a 1-year fellowship. We helped them take nearly a decade of their fellows and assemble a cognitive network. The goal was to predict, with greater certainty than pure chance, how teams could come together to more effectively solve specific problems.
Out of 477,191 possible combinations, we were able to identify two teams with nearly ideal balances of similarity and difference. In this example, there are three people (blue dots) with certain attributes in common and some not (yellow dots). There are also artifacts of their work (grey dots) that have some overlap with each other and that bring some unique perspective.
Capitalizing on the Network
What we’ve learned since then and built into our approach is how to enable this approach on a larger scale. If we only find potential for innovation here and there it’s not that much different than a fortuitous conversation. We want to facilitate innovation systemically.
The major elements of a system that facilitates innovation are metadata, modularity, fluidity, and scale.
Metadata is how we come to understand where overlap can lead to innovation. The common characteristic of having branches that allowed visualization techniques from multiple fields to lead to improved diagnosis of heart conditions. An innovation system must repeatedly analyze and apply that meta-perspective to understand where the overlap is subject to existing in the first place.
Modularity is the need to break artifacts down into elements that can work together. It was a lucky and rare event that an MRI machine could ingest astronomical data. Innovation systems need to facilitate that kind of interoperability intentionally and widely.
Fluidity refers to the ease with which members of a community can experiment with potentially innovative ideas. Systems can stack the odds with data and suggestions, but humans have to experiment to find the new ideas that are actually good. If it’s too painful to experiment, we’ll never try anything like jamming some astronomical data into an MRI machine.
Finally, scale is necessary to achieve a higher likelihood of innovation. More people, attributes, and artifacts increase the breadth and depth of metadata that reveals potential innovation.
More Becomes Less
I am a data scientist and an engineer. I’m trained to seek economy. And as I see it, all of this effort is actually done in the name of creating and innovating less, ironically. Stop being so creative! The solution to your problem, or key elements of it, has probably already been solved in some other form. There’s a way to realize what ideas you can rely on and translate to your field, before you reinvent them.
Comments